Understanding the Importance of Machine Learning Data Annotation in Business
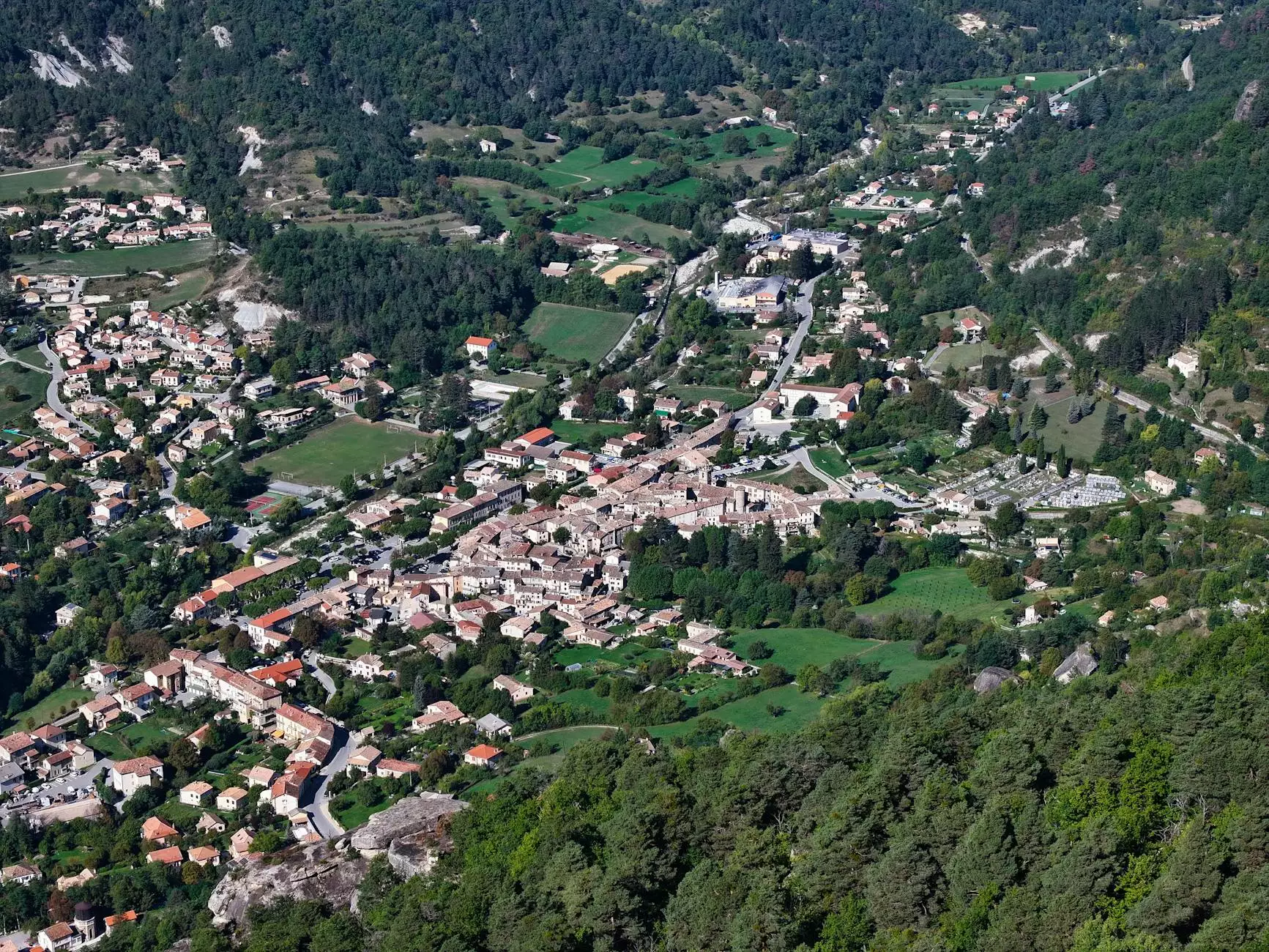
The advent of machine learning has dramatically transformed various industries, particularly in the realms of data analysis and automation. One of the critical components that underpin successful machine learning projects is data annotation. This article delves into the concept of machine learning data annotation, its relevance to businesses, and how it can significantly bolster operations in sectors like Home Services and Keys & Locksmiths.
What is Machine Learning Data Annotation?
At its core, machine learning data annotation is the process of labeling data for training machine learning models. Data can be in various forms, including images, text, audio, and video. By providing accurate labels, data annotation allows machine learning algorithms to learn patterns and make predictions based on new, unseen data.
Types of Data Annotation
- Image Annotation: Involves tagging images with relevant labels, useful in fields like security surveillance and diagnostics.
- Text Annotation: Common in natural language processing (NLP), where entities, sentiments, or specific sections of text are tagged.
- Video Annotation: Labels frames in videos, crucial for applications in security and sports analytics.
- Audio Annotation: Tags audio clips for speech recognition and other audio-related applications.
Why is Machine Learning Data Annotation Essential for Businesses?
The necessity of data annotation in machine learning cannot be overstated. Here’s why it is particularly vital for businesses looking to leverage machine learning technologies:
1. Enhancing Decision-Making Processes
By using accurately annotated data, businesses can make informed decisions based on predictive analytics. Machine learning models can identify trends, customer behaviors, and operational efficiencies, thus assisting companies in strategizing effectively.
2. Improving Customer Service
In sectors such as Home Services and Keys & Locksmiths, understanding customer preferences is crucial. Machine learning algorithms trained on accurately annotated data can help predict customer needs and enhance the overall service experience.
3. Automation of Routine Tasks
Automation through machine learning reduces the burden of repetitive tasks. For example, in locksmith services, data-driven solutions can help automate scheduling and inventory management based on previously annotated data patterns.
The Role of Data Annotation in Keymakr.com’s Business Model
Keymakr.com is a business that operates in the Home Services and Keys & Locksmiths sectors. Here’s how machine learning data annotation can revolutionize their operations:
Optimizing Service Delivery
Using annotated data, Keymakr.com can create models that predict peak service times, customer preferences, and even optimal pricing strategies. By understanding when customers are most likely to require services, Keymakr can ensure they are adequately staffed and prepared to meet demand.
Personalized Marketing Strategies
The wealth of customer data available can be harnessed to create personalized marketing campaigns. By analyzing patterns in customer behavior through annotated data, Keymakr.com can tailor its offerings, cater to specific demographics, and enhance customer loyalty.
Enhanced Security Solutions
Data annotation can also play a crucial role in improving the security services offered by Keymakr.com. By leveraging image and video annotation, the business can develop better surveillance systems, providing customers with peace of mind and improving overall safety.
Challenges in Machine Learning Data Annotation
Despite its benefits, machine learning data annotation comes with challenges:
- Quality Control: Ensuring data quality is paramount. Mislabeling can lead to inaccurate model predictions.
- Resource Intensiveness: Annotation can be time-consuming and requires human resources or specialized tools.
- Scalability Issues: As the volume of data increases, scaling annotation efforts without losing quality becomes challenging.
Best Practices for Effective Data Annotation
To achieve optimal results in machine learning data annotation, businesses should adhere to several best practices:
1. Define Clear Annotation Guidelines
Creating a comprehensive guideline ensures that all annotators understand what is required, leading to consistency and precision in data labeling.
2. Employ Skilled Annotators
Using trained professionals who comprehend the nuances of the data they're annotating can drastically enhance the quality of the annotated data.
3. Utilize Annotation Tools
Investing in advanced data annotation tools can streamline the process, improve efficiency, and reduce time to market.
4. Regular Quality Audits
Conducting routine checks on annotated data helps maintain high-quality standards and address any errors promptly.
Utilizing Technology to Enhance Data Annotation
Innovations in technology, particularly software solutions powered by AI, are optimizing the data annotation process. Automated tools can assist in accelerating the annotation while maintaining quality, allowing businesses to focus on core operations.
- Automated Video Annotation: Utilizing AI to automatically label video frames accelerates the process and increases accuracy.
- Natural Language Processing Tools: For text annotation, AI can help identify and label entities, sentiments, and relations in text.
- Image Annotation Tools: Software that can auto-annotate images based on pre-defined patterns can save time and resources.
Conclusion: The Future of Machine Learning Data Annotation
The role of machine learning data annotation in enhancing business operations cannot be ignored, especially within the Home Services and Keys & Locksmiths sectors. As businesses like Keymakr.com look to harness the power of data, investing in quality data annotation processes will pave the way for improved customer experiences, optimized service delivery, and streamlined operations. By understanding the significance of data annotation and adhering to best practices, businesses can effectively leverage their data to drive growth, innovation, and success in an increasingly competitive market.
In conclusion, as machine learning continues to expand, those companies that prioritize effective data annotation will find themselves at the forefront of their industries. They will not only improve operational efficiency but also unlock new avenues for customer engagement and service excellence.