Understanding Bounding Boxes in Data Annotation: A Comprehensive Guide
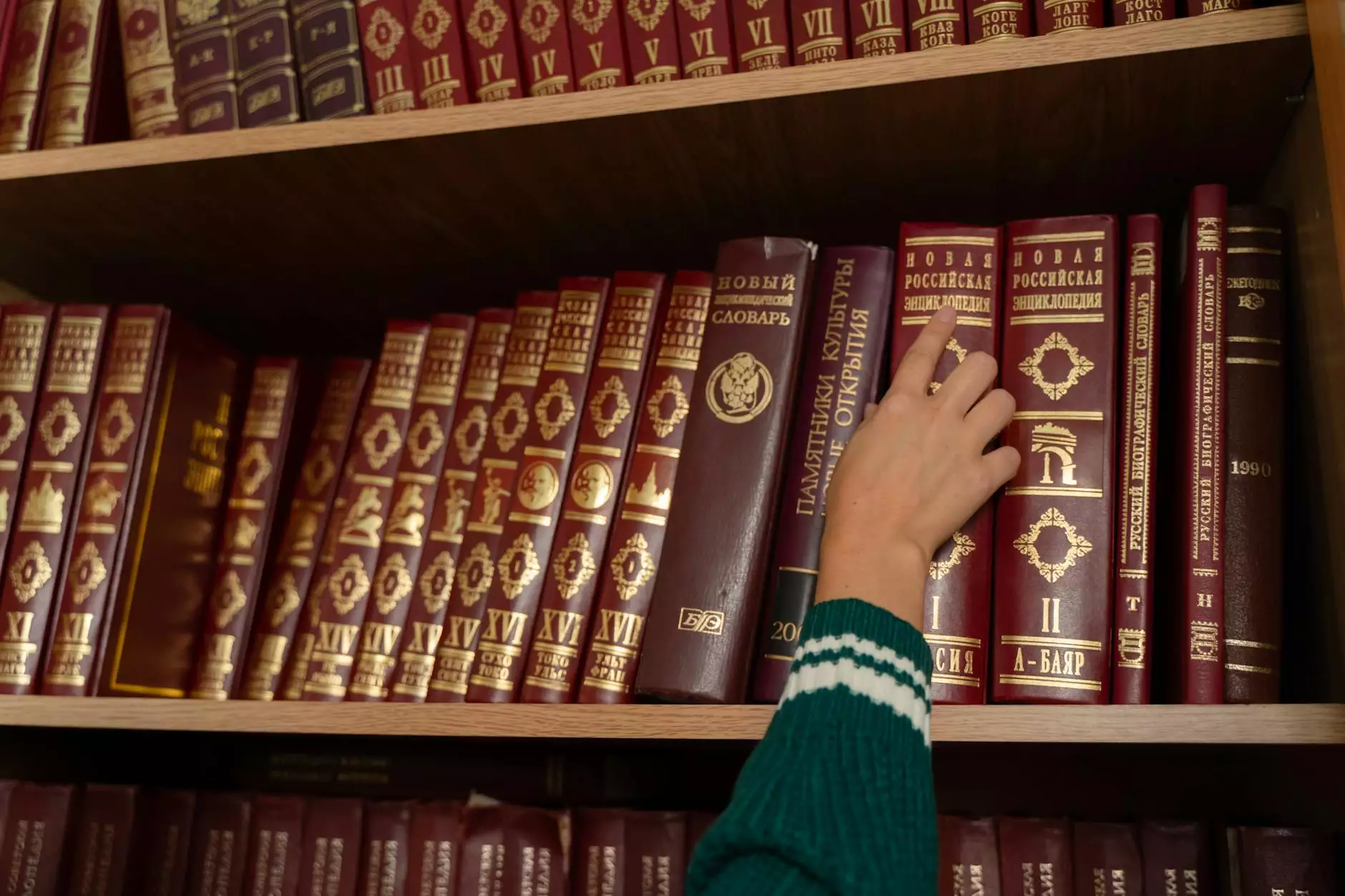
In the fast-evolving world of artificial intelligence and machine learning, bounding boxes have emerged as a fundamental concept, particularly in the realm of data annotation and image processing. As businesses strive to harness the power of data to optimize their operations and improve their products, understanding the significance of bounding boxes is essential. This article delves into the importance of bounding boxes, their applications, and how businesses can leverage them for better data annotation practices.
What are Bounding Boxes?
A bounding box is defined as a rectangular area that encapsulates an object of interest within an image. This concept is particularly prevalent in computer vision, where algorithms are trained to detect specific objects, making it crucial for tasks like object detection, face recognition, and image segmentation.
Key Properties of Bounding Boxes
- Rectangular Shape: Bounding boxes are typically rectangular, allowing for straightforward mathematical calculations.
- Coordinates: Each bounding box is defined by its coordinates, usually represented by the top-left corner and the bottom-right corner (x1, y1, x2, y2).
- Dimension Specifications: The width and height can also be specified, giving a comprehensive view of the area covered.
Why Are Bounding Boxes Important?
Bounding boxes are crucial for numerous reasons, especially in the data annotation landscape:
1. Object Detection
Object detection algorithms rely heavily on bounding boxes to identify and classify objects within an image. Each bounding box provides a predictor with the information necessary to understand where an object is located, enabling the algorithm to make accurate predictions.
2. Image Annotation
In image annotation, bounding boxes help in labeling images by marking the boundaries of objects, guiding machine learning models in learning from labeled datasets.
3. Training Data Creation
For machine learning models to perform effectively, they must be trained with high-quality datasets. Bounding boxes play a vital role in creating these datasets by providing a clear definition of where objects are located within training images.
Applications of Bounding Boxes in Business
Businesses across various sectors have started to recognize the significance of bounding boxes. Here are some of the most notable applications:
1. Autonomous Vehicles
In the automotive industry, bounding boxes are employed in the development of self-driving cars. These systems utilize computer vision algorithms to detect and classify surrounding objects, such as pedestrians and other vehicles, by using bounding boxes.
2. Retail and Inventory Management
Retail businesses utilize bounding boxes in image-based inventory management systems. By integrating computer vision with bounding boxes, businesses can automate stock checks and monitor product placements on shelves.
3. Healthcare Diagnostics
In healthcare, bounding boxes are increasingly used in diagnostics tools for analyzing medical images. They assist in identifying abnormalities in X-rays or MRIs, allowing for quicker and more accurate diagnoses.
How Bounding Boxes Enhance Data Annotation Techniques
Effective data annotation is critical for the success of machine learning projects. Below are ways bounding boxes enhance these techniques:
1. Improved Accuracy
The use of bounding boxes leads to more accurate annotations. By providing clear definitions of the objects’ extent within images, annotators can minimize uncertainty and errors in labeling.
2. Consistency in Labeling
Organizations can achieve consistency in their labeling efforts by standardizing the use of bounding boxes. This helps in maintaining a uniform quality of data across different projects and teams.
3. Time Efficiency
Annotating data with bounding boxes can significantly reduce the time required for manual labeling tasks, especially when using automated tools that integrate AI and machine learning algorithms.
Implementing Bounding Boxes: Best Practices
When implementing bounding boxes in data annotation, consider adopting the following best practices:
1. Define Clear Guidelines
Establishing clear guidelines for how bounding boxes should be drawn can improve the quality of annotations. Specify the level of detail required and annotate edge cases for consistency.
2. Utilize Advanced Annotation Tools
Leverage advanced data annotation tools that offer features like automatic bounding box generation, which can speed up the process and maintain accuracy.
3. Continuous Training
Regularly train annotators on the latest developments in object detection and bounding box strategies to ensure high-quality data annotation.
The Future of Bounding Boxes in AI and Data Annotation
The landscape of artificial intelligence and machine learning continues to evolve, and so does the concept of bounding boxes. Future advancements may involve:
1. Integration with Deep Learning
With the rise of deep learning, the role of bounding boxes may expand further, enabling complex models to learn directly from the structured orientation provided by these boxes.
2. Automated Bounding Box Generation
Improved algorithms could lead to more automated approaches for bounding box creation, dramatically reducing human effort while maintaining high accuracy.
3. Real-Time Data Annotation
There is potential for developing real-time data annotation solutions that utilize bounding boxes for dynamic environments, such as in video analytics and surveillance systems.
Conclusion
In conclusion, bounding boxes are a cornerstone of data annotation and image processing, fundamentally shaping how businesses approach machine learning tasks. From enhancing accuracy to improving efficiency, the relevance of bounding boxes in fields such as autonomous vehicles, retail management, and healthcare diagnostics cannot be overstated. By adopting best practices and staying ahead of advancements in this technology, businesses can optimize their data annotation strategies and harness the full potential of artificial intelligence.
For organizations like KeyLabs.ai, focusing on high-quality data annotation products like those involving bounding boxes can lead to significant competitive advantages in the rapidly advancing world of AI. The importance of quality data cannot be understated, and understanding how to effectively implement and utilize bounding boxes will ensure success in future endeavors.